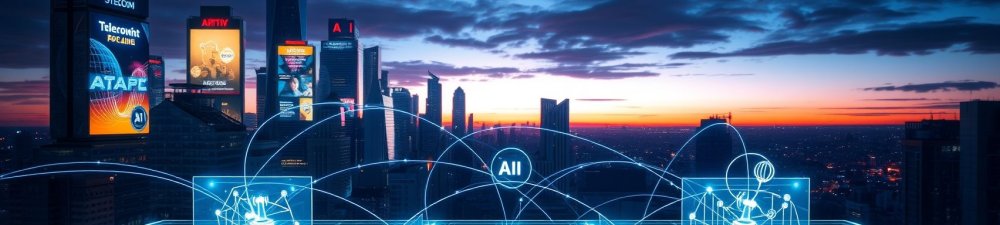
Using AI to Simulate Telecom Signaling Scenarios: A Game-Changer for Engineers and Architects
In today's rapidly evolving telecommunications landscape, the ability to simulate and optimize signaling scenarios is crucial for ensuring robust, efficient, and scalable networks. Telecom engineers, designers, and architects are increasingly looking for innovative methods to enhance their signaling strategies - and artificial intelligence (AI) is emerging as an indispensable tool in this endeavor.
Understanding Telecom Signaling Scenarios
Before delving into the integration of AI, it's essential to grasp what telecom signaling scenarios entail. At its core, signaling is the process that enables network nodes to communicate with each other, coordinating connections and managing the flow of information over the network. It involves protocols such as SIP (Session Initiation Protocol), SS7 (Signaling System No. 7), and various others tailored for mobile and internet networks.
Successful signaling is foundational to delivering quality telecommunications services. It impacts everything from call setup times to the ability to manage complex services like conference calling or video streaming. Given the complexity of modern networks, along with the diverse range of devices and applications, engineers face immense challenges in designing signaling mechanisms that are both reliable and efficient.
The Role of AI in Simulation
Artificial Intelligence offers a revolutionary approach to modeling and simulating these telecom signaling scenarios. Here's how AI is changing the landscape:
-
Predictive Modeling: AI can analyze historical signaling data to develop predictive models that forecast network behavior under different scenarios. By simulating various traffic loads and user behaviors, engineers can anticipate potential issues such as bottlenecks or service interruptions before they impact actual users.
-
Dynamic Simulations: With AI-powered tools, engineers can create highly dynamic simulations that adapt in real-time to changes in network conditions. This adaptability allows for testing various signaling protocols against fluctuating network demands, enabling engineers to optimize performance proactively.
-
Automated Testing: Traditional testing of signaling scenarios can be time-consuming and resource-intensive. AI can streamline this process through automation, executing complex test cases across diverse signaling parameters without human intervention. The increased speed and accuracy of testing results in a more reliable signaling infrastructure.
-
Anomaly Detection: AI algorithms can identify patterns in signaling data, making it easier to pinpoint anomalies or irregular behaviors indicative of network faults. By implementing machine learning models, engineers can ensure early detection of issues, facilitating quicker troubleshooting and resolution.
-
Scenario Generation: One of the most powerful aspects of AI is its ability to generate diverse signaling scenarios based on numerous variables. By considering factors such as network topology, traffic patterns, and user behavior, AI can create a wide range of scenarios for comprehensive testing. This thorough approach helps in understanding how different signaling approaches may perform across varied conditions.
Bridging the Gap with Automation Tools
While the benefits of AI in simulating telecom signaling scenarios are abundant, the real challenge lies in implementation. This is where platforms like vpost.nl come into play. Vpost offers automation solutions designed specifically to help telecom engineers, designers, and architects leverage AI for their signaling simulations.
With vpost's tools, telecom professionals can easily automate the generation of signaling scenarios and integrate predictive modeling into their workflows. The interface is user-friendly, allowing engineers to focus on critical design decisions rather than getting bogged down with manual testing processes. This allows for greater efficiency and accuracy in network design and optimization efforts.
Conclusion
The incorporation of AI into the simulation of telecom signaling scenarios marks a paradigm shift for engineers and architects. By harnessing the power of machine learning and dynamic modeling, professionals in the telecommunications field can optimize network performance, enhance reliability, and ultimately deliver superior service to end-users.
As the telecom landscape continues to evolve, embracing AI technologies and automation tools like those offered by vpost.nl will be imperative for staying competitive. The future of telecommunications signaling is here, and by leveraging these resources, professionals can unlock new levels of network efficiency and innovation.
By investing in such technologies, telecom engineers can ensure they are not only prepared for today's challenges but also equipped for the future's demands.